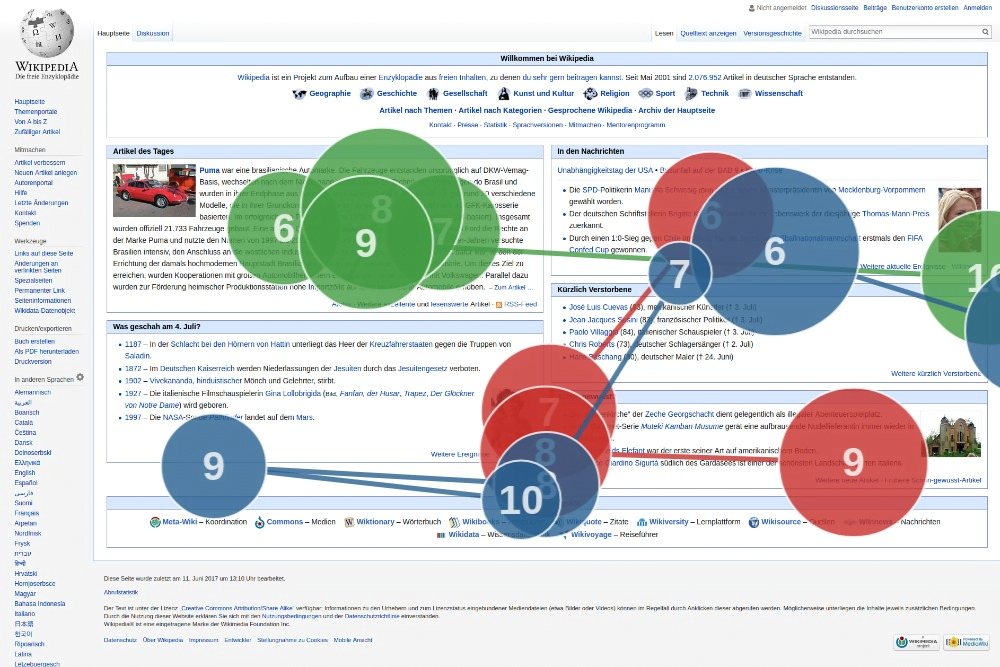
Predicting consumer choices from eye tracking data
- Post by: Jungpil Hahn
- February 10, 2023
- No Comment
This morning we had a fascinating talk by Alex Tuzhilin (NYU) on his recent work entitled “Predicting Consumer Choice from Raw Eye-Movement Data Using the RETINA Deep Learning Architecture” (the paper is available on SSRN: https://ssrn.com/abstract=4341410) with his colleagues Moshe Unger (Tel Aviv University) and Michel Wedel (University of Maryland).
The essence of his talk was that it was possible to utilize advanced deep learning (Dl) techniques (transformer + metric learning) with “raw” eye-tracking data (i.e., just the x-y coordinates of the eye gaze without any additional contextualizations including area of interest (AOI) or content semantics) to predict consumers’ product choices (on a webpage that showed four products in a consideration set along with their specifications) with higher accuracy than conventional state-of-the-art machine learning approaches calibrated with fixation data (which includes raw gaze data, scanpath data, AOI data and image data). There were three additional surprising results — 1. the proposed DL model with only raw gaze data did NOT require a huge dataset — the superior performance was demonstrated with only 112 subjects’ eye-tracking data, 2. prediction reached very good accuracy very fast — we don’t need to observe the full interaction with the webpage to predict the product choice; at least in their experiment, product choice could be accurately predicted after about 5 seconds of subjects perusal of the webpage where the average total duration for the choice task was around 10 seconds, and 3. the SHAP analysis of the AutoML models showed that among the different types of data (raw, fixation, AOI and image), raw gaze data had the highest overall importance.
The study in and of itself was very interesting. But to me what was more interesting were the implications of such technologies.
First, if it is possible to accurately (maybe not perfectly but with sufficiently high accuracy) predict consumers’ product choices, wouldn’t it be possible to rearrange / redesign webpages strategically to make (or at least strongly nudge) consumers to choose a preferred product (e.g., perhaps one with the greatest profit margin, or ones with excess inventory, etc.) Such technology could easily lead to anticompetitive behaviors especially when large platforms control the display of product offerings. I am reminded of the US Department of Justice’s antitrust investigation into SABRE in the 1980s. Will these technologies also need to be regulated? If so, how? What is acceptable nudging behavior? Isn’t marketing and advertising pretty much the same thing? How can we clearly distinguish between simple nudges and manipulation (i.e., loss of the exercise of free will in choices)?
Second, although the study used eye-tracking data collected using specialized infrared eye tracking machine, the rate at which webcams are improving in quality and resolution along with more research in the Computer Vision area in inferring coordinate gaze data just by looking at someone’s eyes, makes me nervous that such technologies can quickly be deployed at large scale and technology companies/platforms that control the data interface (e.g., cell phone manufacturers since most phones have front-facing cameras; video conferencing providers such as Zoom, AR/VR headset manufacturers etc.) will gain immense power. For example, its not difficult to imagine analyzing individuals gazes during zoom meetings to predict behaviors (e.g., negotiation strategies/outcomes) and value-added services can be offered to productize strategic insights in real time.
Third, there is definitely a “creep” factor but I’m wondering what the legal norms might be for evaluating such technologies. Advanced AI techniques are just being used to create accurate prediction models about human behaviors. But isn’t generating insights about human behaviors simply psychology research (I know I am oversimplifying)? How is this application of AI and eye tracking data different from using theories derived from psychology laboratory studies to create more favorable (profitable) setups? For example, is the widely adopted practice of the decoy effect a problem? Perhaps, the difference is based on the general behavior vs. a specific person’s behavior. Psychological theories offer us a general understanding of behaviors whereas the kinds of AI techniques used in this study give the user of such technologies predictions about specific / targetted individuals.
Well, the genie is already out of the bottle. We really need to think about governance mechanisms such that technologies are used safely and for good.
Image credits: Tschneidr @ https://commons.wikimedia.org/wiki/File:Gaze_plot_eye_tracking_on_Wikipedia_with_3_participants.png